Dodgy Data Makes AI Less Useful
Artificial intelligence may be failing thanks to human error according to a new study. That's because data AI models use to learn how to identify images is not always correct to start with.
The problem affects neural networks, which are designed to work in a similar way to the human brain, considering multiple possibilities at the same time. The idea is to get the benefits of human thought but with the speed and reliability of computers.
In principle, training these AI models is a straightforward process. Rather than humans creating a set of rules for the models to follow, they simply give them a large data set of labeled images and let them figure out their own rules for how to identify different objects. Similar processes work on other data such as audio clips and passages of text.
Ambiguous Pictures
The problem, according to the MIT research, is that these data sets often have errors in the human-created labels. They calculated that 3.4 percent of the data labels they examined were either flat-out wrongly labeled or were questionable in some way. (Source: theregister.com)
One example of the latter is an image showing a bucket filled with baseballs and labeled with only one word. Either "bucket" or "baseballs" would make sense to a human but the choice could affect the lessons the AI learns from the information.
Other cases came down to whether people took a literal approach or concentrated on what was significant about an image. For example, an image looking down at a set of circular steps prompted a divide about whether it's primary label should be "lighthouse" or "coil". (Source: labelerrors.com)
Manual Review Flawed
Ironically tech company attempts to deal with such inaccuracies may be making things worse. The Register notes that some companies used low-paid outsourced workers to review the data sets and spot errors.
The problem was that the system they used to assess the performance of these workers assumed that those who picked up a lot of apparent errors were themselves either getting things wrong or deliberate trying to sabotage the system. The workers figured this out and became much more likely to "agree" the original label was correct rather than say what they actually believed.
What's Your Opinion?
Is this a big problem for AI? Have you come across similar mislabeling? Is there a simple answer or is this an inevitable issue with multiple ways to interpret an image?
Most popular articles
- Which Processor is Better: Intel or AMD? - Explained
- How to Prevent Ransomware in 2018 - 10 Steps
- 5 Best Anti Ransomware Software Free
- How to Fix: Computer / Network Infected with Ransomware (10 Steps)
- How to Fix: Your Computer is Infected, Call This Number (Scam)
- Scammed by Informatico Experts? Here's What to Do
- Scammed by Smart PC Experts? Here's What to Do
- Scammed by Right PC Experts? Here's What to Do
- Scammed by PC / Web Network Experts? Here's What to Do
- How to Fix: Windows Update Won't Update
- Explained: Do I need a VPN? Are VPNs Safe for Online Banking?
- Explained: VPN vs Proxy; What's the Difference?
- Explained: Difference Between VPN Server and VPN (Service)
- Forgot Password? How to: Reset Any Password: Windows Vista, 7, 8, 10
- How to: Use a Firewall to Block Full Screen Ads on Android
- Explained: Absolute Best way to Limit Data on Android
- Explained: Difference Between Dark Web, Deep Net, Darknet and More
- Explained: If I Reset Windows 10 will it Remove Malware?
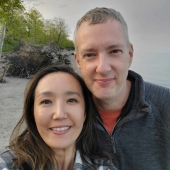
My name is Dennis Faas and I am a senior systems administrator and IT technical analyst specializing in cyber crimes (sextortion / blackmail / tech support scams) with over 30 years experience; I also run this website! If you need technical assistance , I can help. Click here to email me now; optionally, you can review my resume here. You can also read how I can fix your computer over the Internet (also includes user reviews).
We are BBB Accredited
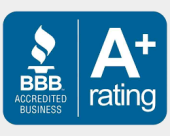
We are BBB accredited (A+ rating), celebrating 21 years of excellence! Click to view our rating on the BBB.
Comments
You get what you pay for
GIGO
On the one hand, AI is exciting.
On the other, AI is frightening.
In "The Runaway Robot" Lester Del Rey postulated (through Captain Becker) that some day, man would build a robot "smarter" than himself.
I just hope it has an OFF switch.